by Kim Reid
I thought I had a
I spent the next two days downloading and analysing the data only to find a result that looked like this.
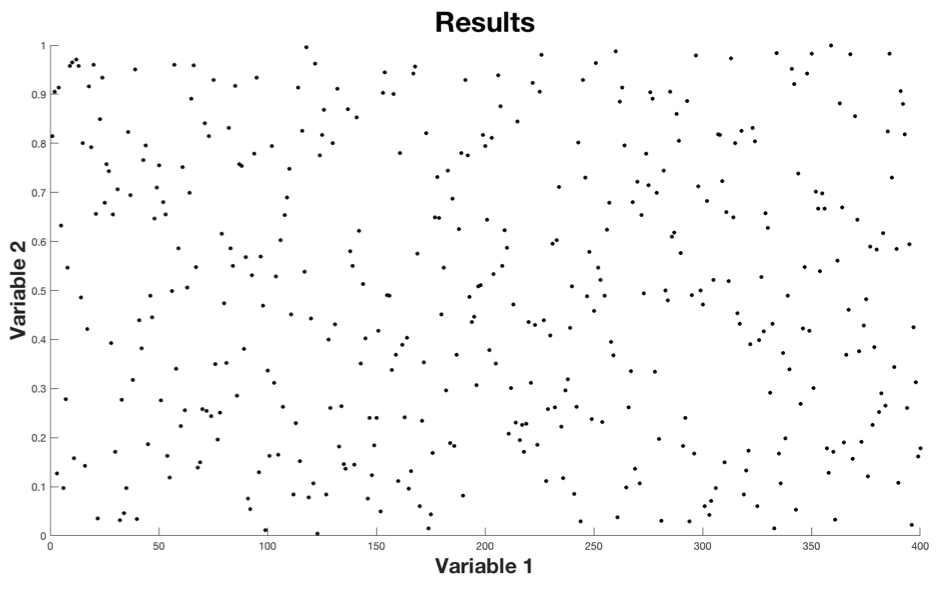
Zero relationship between the variables. I may as well have used a random number generator like I did to make the above image.
The Stages to Acceptance
Like the first stage of grief, the first stage of a null result is shock. “What!” one might exclaim, followed closely by denial, “that’s not supposed to happen!”.
You might replot or recalculate the result assuming there is simply a typo in the code until you realise the code is flawless, and your hypothesis is wrong.
Bargaining happens next. That’s when you tilt your head sideways and mess with the domain of the data until a trend appears before guilt takes over reminding you not to cherry-pick.
The fifth stage is the worst: depression.
A few blocks of chocolate later, and you should arrive at the acceptance stage where your mind is clear again to re-evaluate your original hypothesis and work out why it was wrong.
For the record, it turned out I was taking a spatial average over a highly variable region, hence destroying the signal. After using a different method to test my original hypothesis, I found some neat results.
But, sometimes, there is no mistake and the null result is genuine, which raises an important question. Should we still publish the null result? We publish results that move the field forward, but for every step forward, there are at least two steps side-ways. Publishing null results could prevent scientists repeating experiments, but it could also be a huge time drain.
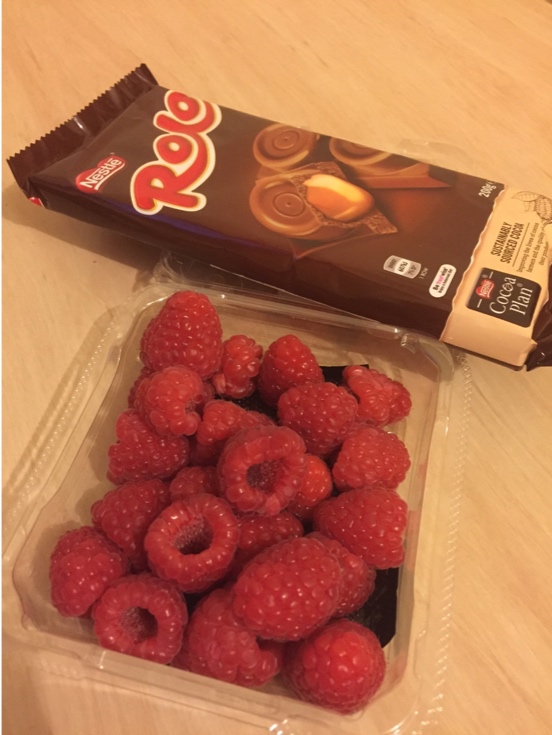