Accurate quantification of land degradation is a global need, particularly in the world’s dryland areas. However, there is a well-documented lack of field data and long-term observational studies for most of these regions. Both the rainfall and vegetation datasets used for land degradation assessment contain errors and uncertainties, but little work has been done to understand how this may impact results.
This study uses six rainfall and two vegetation datasets to perform land degradation detection over Australia. Large differences in the data and methods used to produce the precipitation datasets did not significantly impact results.
On the other hand, the vegetation dataset selection had a much greater impact.
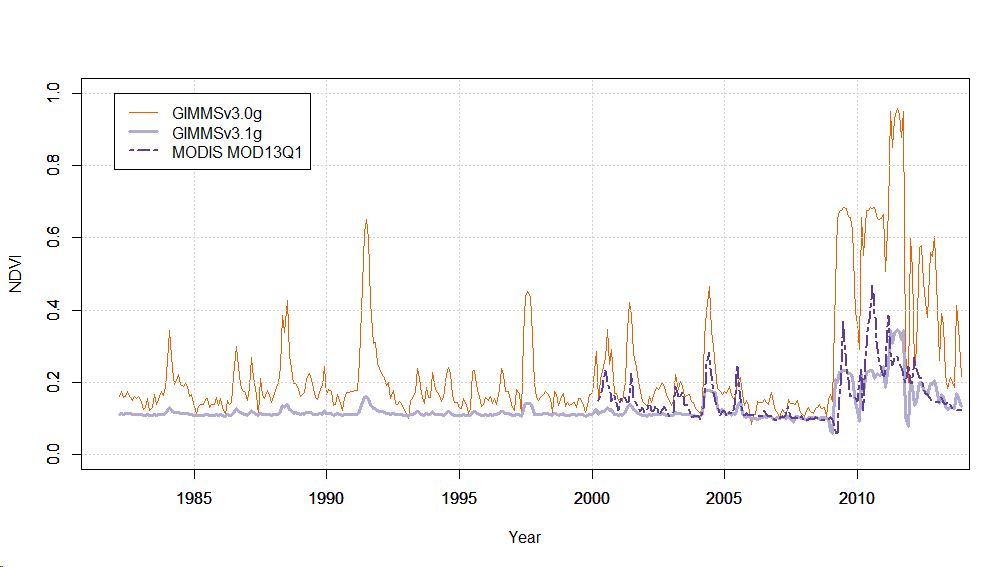
Calibration errors in the widely used Global Inventory Monitoring and Modeling System Version 3 NDVI (GIMMSv3.0g) dataset caused significant errors in the trends over some of Australia’s dryland regions. Though identified over Australia, the problematic calibration in the GIMMSv3.0g dataset may have affected dryland NDVI values globally. These errors have been addressed in the updated GIMMSv3.1g which is strongly recommended for use in future studies.
This study also showed that using multi-run ensemble made using different input datasets provides more comprehensive quantification of uncertainty and errors in space and time.
- Paper: Burrell, A.L., Evans, J.P., Liu, Y., (2018). The impact of dataset selection on land degradation assessment. ISPRS Journal of Photogrammetry and Remote Sensing 146, 22–37. https://doi.org/10.1016/j.isprsjprs.2018.08.0